Author
David Fehrenbach
David is Managing Director of preML and writes about technology and business-related topics in computer vision and machine learning.
Logistics always strives to deliver operational excellence and customer satisfaction. In the context of construction sites, logistics refers to the efficient coordination and management of various resources, materials, equipment, and information to ensure the smooth flow of activities throughout the construction process. What does the computer vision software from preml have to do with it? Find out more in this text!
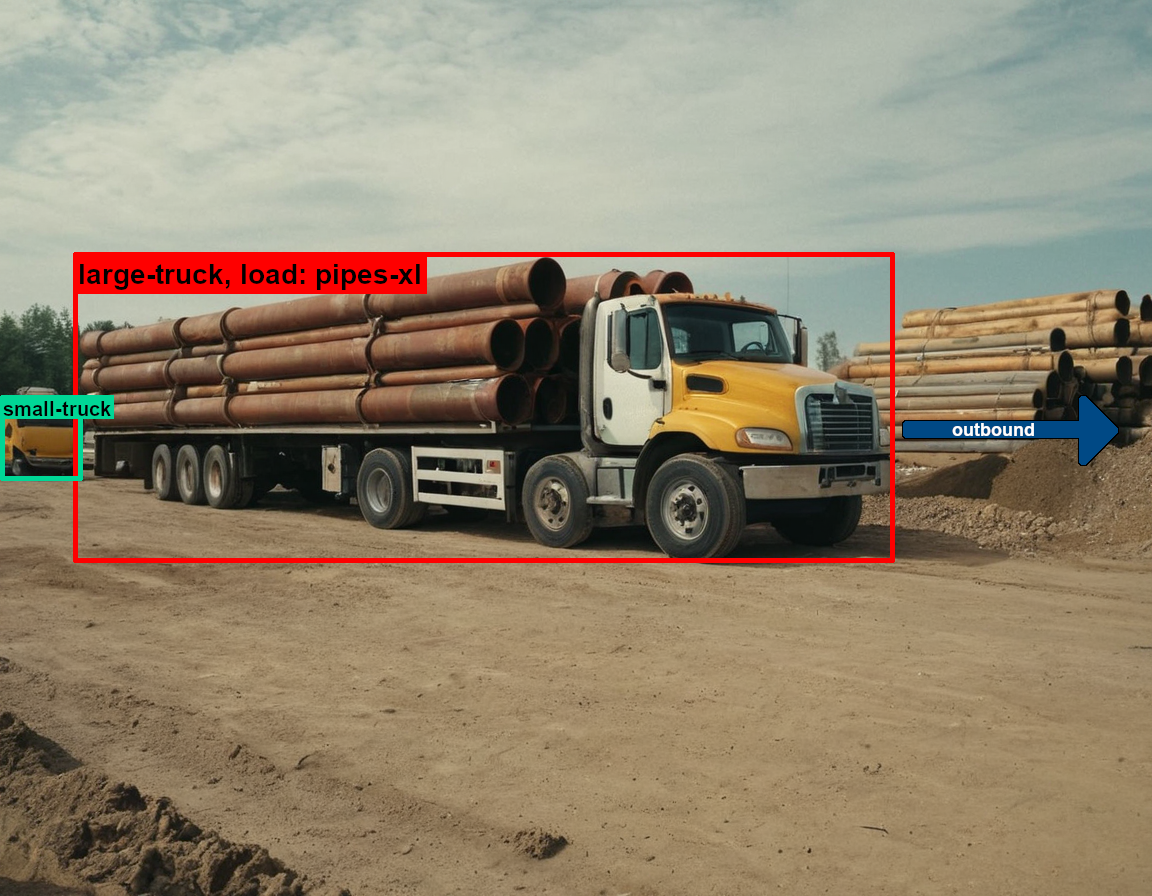
Image 1: Visual project illustration – A bounding box indicates the detection of the vehicle, its drive direction and loading (c) preML GmbH
Problem: Extract Real-Time Information related to Construction Vehicle
Extracting real-time information about the location, arrival time, loading, and driving directions of construction vehicles poses a notably challenging task. Humans often drive and load the vehicles, acting according to their intentions and knowledge. However, this information can be very valuable to systematically automate and optimize the actual building process. Other workers don’t know when the vehicle arrives, what it has loaded, even if it’s currently driving in their direction or not might be unknown. From a technological standpoint, there are solutions to achieve this, utilizing GPS information, barcodes, RFID, Beacons, etc.. However, implementing these solutions in a fleet, particularly in projects with multiple stakeholders or changing subcontractors, is not always straightforward. Additionally, environmental constraints, cost considerations, and the potential for errors can further complicate the adoption of certain technologies. For reasons, in the specific use case our customer opted to collaborate with us.
Approach: Training AI-based Computer Vision Models based on image data extracted from video streams
For a prominent player in the construction business, preML developed a proof of concept (PoC) for software automatically extracting information about place, time and driving direction of vehicles using computer vision. The PoC was conducted in a specific construction setting, and the results are from that setting only, but have potential applicability to other scenarios. In Total 10 standard surveillance video camera streams were collected with the purposes to create a training dataset. After processing, image extraction and automatic pre-selection the customer provided annotations. Afterwards, state-of-the-art computer vision models are used to detect vehicles, driving direction and loading actions. Relevant information which had to be extracted were especially arrival & departure times, driving direction and allocation of load. The model was tested on unseen data and evaluated.
Performance: Quantitative and qualitative evaluation
Quantitative and qualitative results of the PoC are very promising for the specific settings tested. The model is very strong in detection of vehicles, counting, and drive direction estimation in the setting. However, when it comes to load determination, there might be still improvement in the current setting, mainly delete possible by increasing the number of training data for the model and optimizing the camera position. The PoC phase was evaluated as successful by the customer and further cooperation in the form of a rollout is planned for this year.
Sounds interesting? Contact us for more!
We look forward to publishing more results from the project soon!
David
Feel free to contact us anytimes at contact@preml.io
Autor
David Fehrenbach
David is Managing Director of preML and writes about technology and business-related topics in computer vision and machine learning.